The tracking of movements and poses is a central field of application for artificial intelligence in the future. With the macro trend of gesture control on the rise and the increasing importance of machine learning in the interpretation of human behavior, new areas of application for the interpretation of poses are needed.
The areas of application are manifold: from mixed reality, the recognition of sign language, control through whole-body gestures and even the quantification of exercises in sports, anything is conceivable. In her latest blog entry, Google has now presented her current research successes.
BlazePose, as the new method is called, is used to interpret body poses and to document and evaluate the various movements by means of machine learning. Unlike current pose models that are already used in AI, BlazePose locates much more precise key points on the human body. This makes it perfect for fitness applications and their interpretation. One potential application is in smart mirrors, for example. These, installed in your home gym or fitness studio, can automatically and accurately record the successful execution of exercises and poses and provide individual suggestions for improvement.
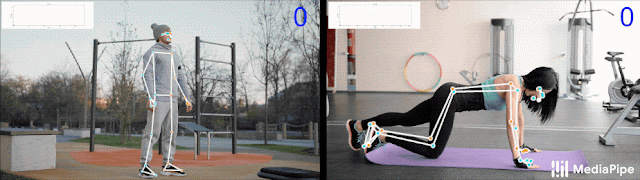
However, to optimize the use of BlazePose, the Machine Learning Pipeline requires an extremely high reaction speed between pose detection and tracking. This should be a few milliseconds per frame. To make this possible, Google has been looking for the best identification point for artificial intelligence. As the strongest identifying feature for AI, they finally identified the user’s face. This has high-contrast features and at the same time varies relatively little in appearance. With the face as an anchor, Google can use the face recognition software to orient itself, which can analyze data within milliseconds. With Facial Recognition as a proxy for the new mail detector, a completely new type of tracking was made possible.
Google sees several applications for BlazePose. For example, they want to build fitness or yoga trackers that can check the quality of the exercises performed in real time.
More details about how the new ML software works can be found here in the Google Blog.